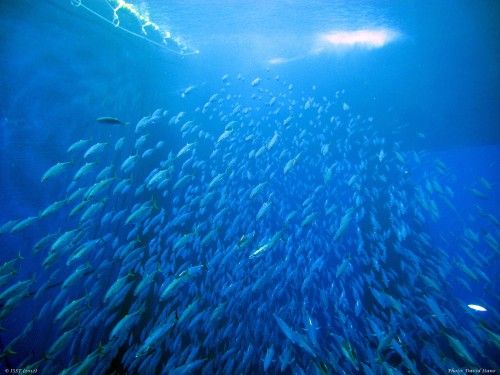
Closing the Data Gap
One of the challenges in knowing how to optimally manage tuna stocks globally is the difficulty of obtaining reliable data for many species. The availability and quality of different types of data vary between species, fleets, and regions.
Stock assessment scientists — on whom fisheries managers rely for accurate and up-to-date stock information — need reliable, high-quality data, because misleading information can easily introduce bias.
Why Stock Assessments?
Before learning more about the challenges in assessing tuna stocks, it is useful to understand why and how a fishery’s stocks are assessed in the first place.
Stock assessments are key to marine resource management. Ideally, they provide high-quality science information to fisheries managers to answer important questions like:
- Is the stock experiencing overfishing or is it overfished?
- If a stock becomes depleted, what steps are required to rebuild it to healthy levels of abundance?
- Are the methods used to catch the tuna also catching significant numbers of non-targeted species?
Answers to these questions help managers at the world’s tuna regional fisheries management organizations (RFMOs) make the best decisions to ensure sustainable fisheries, healthy ecosystems, and productive coastal communities.
Acknowledging the Operational Data Gap
An important constraint in assessing tuna stocks is their sheer size. Tuna stocks cover such vast areas that fishery-independent surveys are rarely cost effective.
As a result, stock assessments rely on data such as catch, effort, and size information, which must be obtained from the fleets and fishing vessels themselves, via logbooks, fishery observers, and sampling both onboard vessels and in port. Stock assessments rely on long-time series of data, so any changes in the way data are collected can also make life difficult for stock-assessment scientists.
Many tuna-stock assessments rely heavily on catch rate (or catch-per-unit-of-effort, or CPUE) data, which are collected by individual fleets. Albacore, bigeye and yellowfin tuna assessments especially depend on longline CPUE data. Yet the quality and completeness of CPUE data, fleet to fleet, vary enormously. Some fleets can only provide data for a short period and a relatively small area, whereas others are more widespread. The Japanese longline fleets, in particular, have logbooks and size data for many stocks that go back to the 1950s and 60s.
Before they can be used in an assessment, CPUE data need to be “standardized.” A statistical analyst runs models that adjust for factors such as fishing area, season, and targeting method, and provide indices of relative stock abundance over time. CPUE standardization is more reliable when based on detailed information about each set, which is called “operational” data.
Ideally, the data will include collective information from all fishing fleets. But many tuna RFMOs do not require the fleets in their member countries to submit operational data. Without data-sharing arrangements, individual scientists from those countries can analyse data only for vessels flagged to their country.
And Understanding Its Impact
As a result, there can be multiple indices from different countries just for one stock. When two indices show conflicting abundance trends, they cannot both be correct, so stock-assessment analysts must weigh up the evidence for each index and identify what might have caused the differences. This can be difficult when the original datasets are not available to explore and check.
In addition, each individual fleet may convey only part of the bigger fishing picture. Fleets may fish in different parts of the ocean and move around through time. Datasets start in different years, and some fleets change their fishing practices.
Scientific Collaboration to Improve Data
Given these data-reporting inconsistencies, and the problem of having multiple abundance indices, organizations like ISSF are invested in helping to improve the accuracy and uniformity of fisheries data.
In 2015, for example, ISSF provided funding for a joint standardization of bigeye and yellowfin CPUE data in the Indian Ocean. This collaborative analysis was proposed by the major longline fishing states Japan, Chinese Taipei and Korea, which made their operational data available. The analysis was a group effort involving scientists from each of those participating member states, and with me as an independent consultant. Similar collaborative work was occurring in other regions, for example, in the Western and Central Pacific Ocean, organized by SPC.
With access to the longest and most spatially distributed dataset available — shared confidentially — we explored the data and developed longline CPUE indices using agreed-upon and fully documented methods. Having all of these fleets participating made it easier for the group to reach a common understanding about how to address each problem that came up during the analysis.
Ultimately, this allowed the CPUE indices to be accepted by all participants, and removed a major obstacle to achieving consensus on bigeye stock status. This process has been repeated for Indian Ocean bigeye, yellowfin, and albacore in subsequent years and continues today in the Indian Ocean, with the Seychelles now joining the other national fleets. The Indian Ocean Tuna Commission (IOTC) now funds the effort. Their Scientific Committee has given the collaborative CPUE analyses high priority, and seeks to extend the work to other IOTC species.
In addition, earlier this year, with funding from International Commission for the Conservation of Atlantic Tunas (ICCAT) and ISSF, I worked with scientists from Japan, Korea, Chinese Taipei, and the United States to carry out a joint analysis for bigeye tuna in the Atlantic Ocean and present the results to the bigeye tuna data preparation meeting, where they were accepted.
The indices were then used as the primary source of abundance information in the bigeye assessment that took place this July and which will be reviewed by ICCAT’s scientific committee in October. The use of joint indices helped get rid of inconsistent CPUE trends, whereas the previous bigeye assessment had been affected by inconsistent trends among different indices.
Next Steps
These joint CPUE analyses have proven successful. A critical next step would be the establishment of a data-sharing mechanism by the fleets and RFMOs, respecting confidentiality, so that similar exercises can be repeated smoothly across all RFMO regions and for all eligible species — including bycatch species such as billfish and pelagic sharks.
In the analyses to date, data have been shared only during the joint meetings. This approach, although a welcome step forward, nevertheless requires considerable international travel, and the brief availability limits the analysis that can be undertaken and increases the risk that errors may affect the indices. An ongoing and secure data-sharing mechanism would both reduce costs and improve the quality and reliability of the results.
With solid scientific data on stock health and abundance, we can assess the impact of fishing effort and RFMO management policy — and propose corrective changes. Is a stock being overfished — or is it rebuilding? Is an RFMO’s harvest strategy appropriate? Do catch limits need to be adjusted? More accurate and comprehensive tuna-stock assessments help us to answer those and other questions — and, ultimately, to safeguard stocks for the future.
Dr. Simon Hoyle works for the National Institute of Water and Atmospheric Research (NIWA) in New Zealand and also is an independent international fisheries consultant. He specializes in quantitative data analysis, fish stock assessment, and population dynamics modeling.
He first developed CPUE indices for tuna stock assessments in 2005, and, since 2015, has been developing joint longline indices in collaboration with scientists from distant water fishing nations.